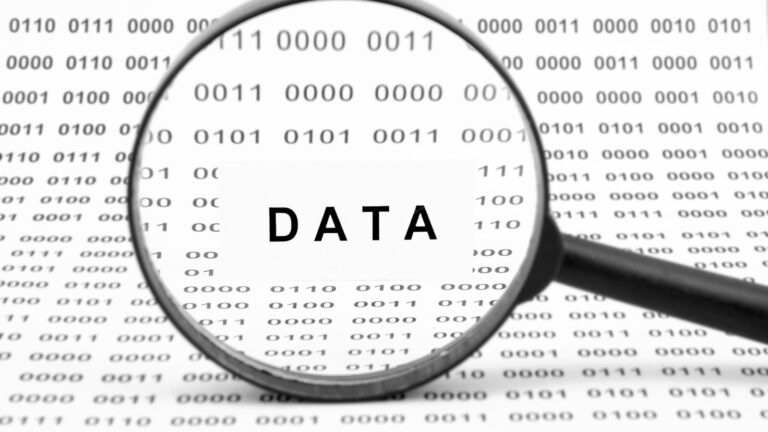
A data lake is a repository that integrates an enormous amount of structured, semi-structured and unstructured data. This means instead of being restricted to storing only structured data, a company can store data in its native format until it needs to be processed and analysed. In the meantime, they are categorised under metadata tags and unique identifiers. As a result, the data is not processed because the purpose of storing the data has yet to be defined.
Data lakes are different from traditional data warehouses, which hierarchically store data, giving precedence to processed and refined information. In other words, data warehouses consist of data already processed for a specific purpose. This article will explain why organisations should move away from data warehouses and adopt data lakes.
Reasons Why You Need a Data Lake
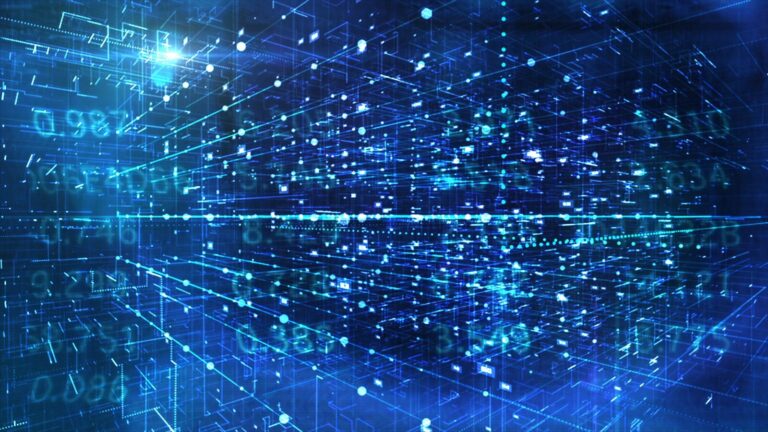
Many companies strive to be data-driven as they can reap the benefits of hidden insights accessed by data analytics. Whether it is increasing their sales, forming better customer relations, optimising daily processors or anything else, a data lake can provide a flexible platform to maximise big data capabilities. Here are four benefits of using a data lake architecture:
In terms of Storage
Due to the enormous number of data stored in the system, data lakes require more storage. This makes data lakes more scalable for large corporations as well as SMEs. Hence, this also makes it a financially feasible solution in the long term. A core feature of the data lake is separating the storage process from the analysis. Hence, where an organisation attempts to upgrade the purpose for which the data is being used, the absence of past data only means that an organisation have already lost integral information. Trying to increase the scope of data analytics in a company will moreover require companies to invest more in storage, making the maintenance of data warehouses comparatively more expensive than maintaining a data lake.
Flexibility
Data warehouses were designed for a specific purpose, making them ideal for static use cases. It would be specialised to perform a certain function to analyse the data and structure it accordingly. While this helps organisations to focus on one primary objective, it also makes it hard to deviate from it. Since data is unstructured in a data lake, the scheme-on-read model provides a flexible architecture to find hidden insights which may not be an organisation’s first thought. In other words, it creates more possibilities for analysis and exploration, allowing advanced technologies such as Artificial Intelligence (AI), Machine Learning (ML), predictive analytics, and others to be integrated into the analysis process. Hence, data lakes are best suited for businesses whose use case tasks change over time.
It Supports Pull and Push-Based Data Integation
Data integration is when a company can access and analyse data from various sources. Since data can be flexibly used for any purpose in a data lake, it supports pull and push-based data ingestion, which refers to two different network communications. While pull relates to situations where information is requested, requiring the server to give the necessary information, thereby ending the exchange, a push-based system provides data over a connection as soon as it becomes available. The latter is similar to receiving notifications or an SMS on a special offer. Data lakes support pull-based ingestion through batch data pipelines and push-based ingestion through stream processing. This makes it possible to use one platform to carry out both processors.
They Are Fast and Secure
Data lakes do not waste time structuring data; instead, they write it on objects or blocks that can be used later for processing. Hence, it can withstand the weight of multiple data sources in real-time. By storing transactional data and historical data in batches, speed is not affected, leading to faster process times and providing a quicker expert analysis output rate. As data lake categorises itself to help make information retrieval much faster, businesses who change their user cases suddenly can easily gain results without much delay. An additional advantage of data lakes is that it is highly secure. Typical data lakes have extra layers of security with multi-factor authentication, offer role-based access and protect all data.
Questions You Should Ask Yourself Before Adopting a Data Lake
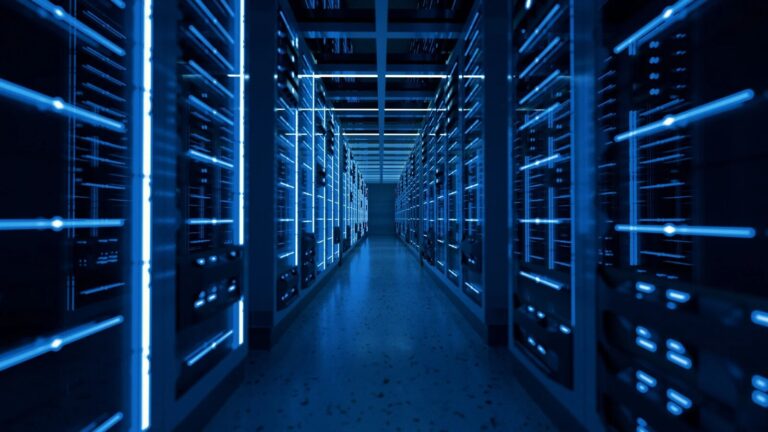
It is important to not consider any architecture as a ‘one-size-fit-for-all’. Popular companies like Google, Amazon and Facebook are prime examples of the adopted data lakes, which helped them create a value chain that offered new business value. This includes finding better advertising methods, improving the speed and quality of their searches, providing them with profitable insights, improving a company’s R&D performance and more. However, throughout the years, many have commented there are drawbacks to storing too much data in a system. One of them is creating data swamps, leading spokespeople in Gartner, for instance, to note that data lake architectures are not the best. Thus, the nature and processes in place in your company will significantly decide whether implementing a data lake is the best approach. Consider the following:
- Do you rely on event-based data? – If an organisation mostly encompasses preprocessed CRM records of financial balance sheets, it makes no sense to adopt a ta lake. However, if the business relies on server logs and requires real-time data, storing data in its raw form and building extract-transform-load(ETL) flows based on the needed use case is better.
- Are you struggling with data retention? – An organisation could find itself in the dilemma of reducing control costs because it is expensive to hold too much data. Instead of choosing between data and reducing costs, a data lake built on inexpensive object storage allows you to have terabytes or petabytes of historical data with no problem.
- Are you focusing on predictable or experimental use cases? – This fundamental question requires companies to ask themselves what they want to do with their data. A data warehouse is more appropriate if the focus is predictable, whereas experimental user cases infused with ML and predictive analytical capabilities will require a data lake.
Cerexio’s Hadoop Ecosystem Supports Data Lake
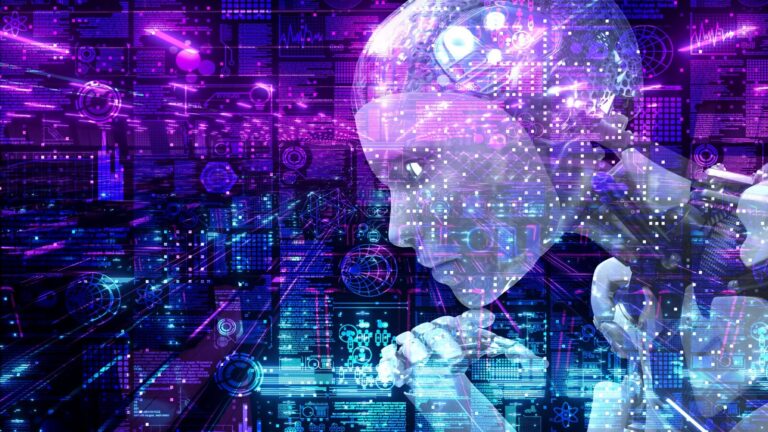
As one of the best software solution vendors in Asia and the world, Cerexio’s solutions are compatible with data lake architectures through its robust Hadoop ecosystems. Cerexio helps you ensure you can meet industrial and commercial practitioners in the industry. Store, process and model data from various devices and receive valuable insights to make intelligent decisions. Unlock the data-tackling capabilities offered by a typical Apache Hadoop ecosystem and enjoy the other advanced features of our digital solutions. They have all equipped with industry 4.0 technologies, including artificial intelligence (AI), machine learning (ML), predictive and prescriptive analytics, digital twin, simulation technology and much more. If you are an SME with futuristic goals, incorporating a scalable digital solution from Cerexio ensures you can easily receive more storage for your corporate data. As it is a license-free software service, this is also a very cost-effective solution in the long term.
Connect with us to learn how the Hadoop ecosystem can help you meet the structural demands of your data specialists.
This article is prepared by Cerexio, a leading technology vendor that offers specialised solutions in the Advanced Manufacturing Technology Sector. The company is headquartered in Singapore and has offices even in Australia. Cerexio consists of a team of experts that have years of experience and holds detailed knowledge on a range of subject matters centric to the latest technologies offered in manufacturing and warehouse operations, as well as in predictive maintenance, digital twin, PLC & instrumentation setup, enterprise integrator, data analytics and total investment system.